Whispers from the AI community
Lights and shadows from the world biggest NLP conference
by Irene Benedetto
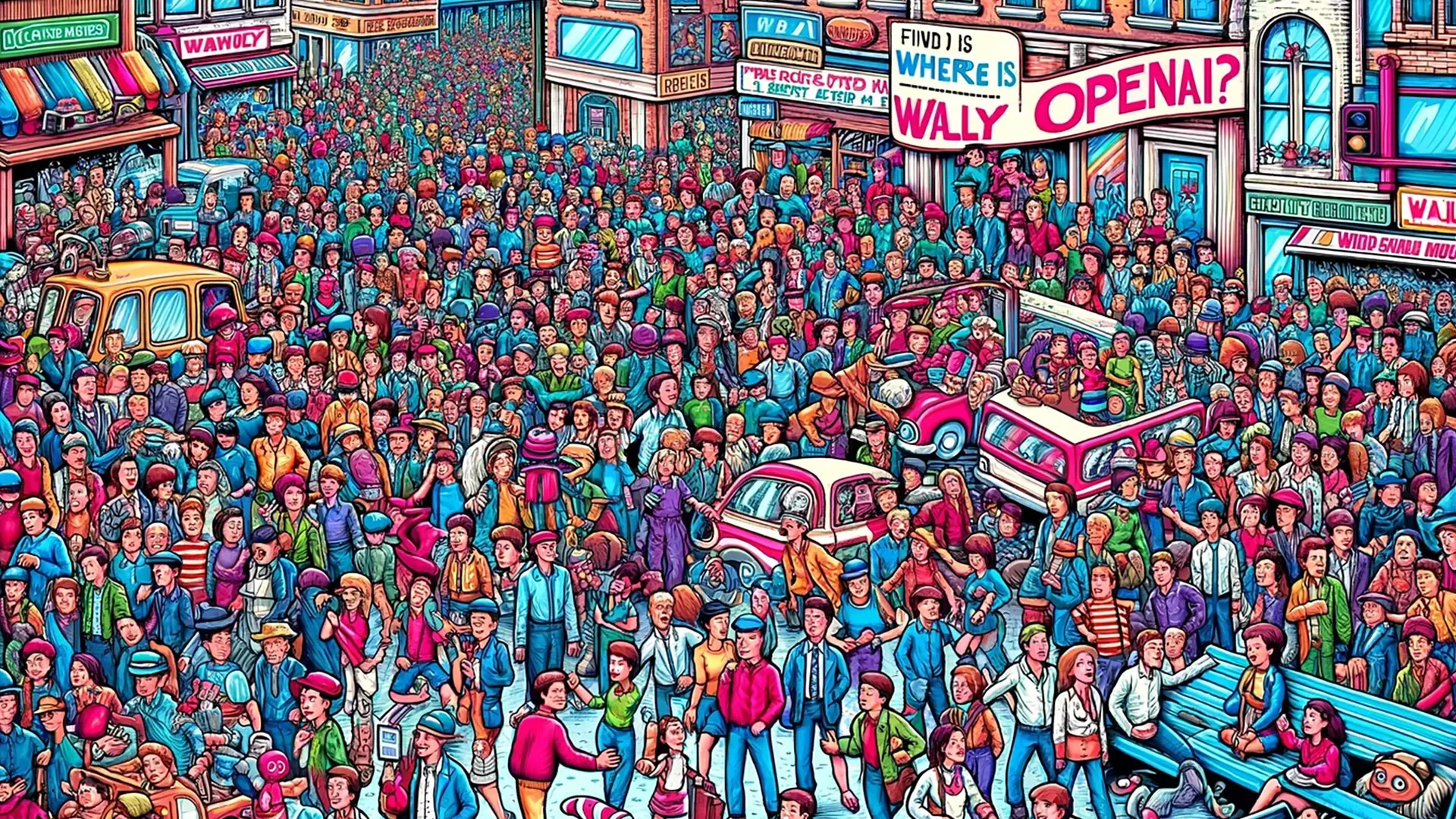
Between July 9 and 14, 2023, companies and researchers from around the world gathered at ACL 2023 (Association for Computational Linguistics) in Toronto. ACL is a conference organized by the leading international organization in the field of Natural Language Processing (NLP). ACL conferences are major events where researchers and practitioners from universities, research centers and companies come together to present and discuss the latest advances in natural language processing.
For those who may not know it, NLP is that branch of artificial intelligence involving language; the one from which ChatGPT originates, for instance.
Just to get an idea on how important and huge — in all senses — this conference is: tier sponsors start from $3,000 and go up to $150,000 for the highest tiers; major sponsors include Meta, Microsoft, Apple, and Google. So, ACL is a big thing, where big companies and top researchers meet to exchange ideas and strike deals. Therefore it is only natural to ask ourselves:
What topics do the biggest players in the industry discuss during ACL? And if AI is really going to have that huge of an impact on our lives, maybe some of the whispers caught during ACL can help us navigate the future?
Hinton's opening keynote
The main opening speech of the conference was given by Professor Geoffrey Hinton. Professor Hinton is among the “Godfathers of Deep Learning”, an extremely significant figure in the field of artificial intelligence and machine learning: his background intertwines cognitive psychology and computer science, and he began working on artificial neural networks in the 1970s and 1980s, when research in this field was in decline. He played a crucial role in rekindling interest in neural networks during a time when AI was way less popular than today. He has received numerous awards for his work, including the prestigious Turing Prize in 2018. In recent years — since he parted ways with Google — he repeatedly warned the scientific community of the potential disastrous consequences of an ubiquitous presence of artificial intelligence in humans’ lives.
Hinton focussed his speech on language models (i.e. algorithms like GPT): language models are trained to predict the next word in the previous context and, according to Hinton, predicting the next word in a language context requires deep semantic understanding. Hinton says that is a capability that both we humans and artificial intelligence-based language models possess.
He insisted that the occasional errors in language patterns produced by AI do not necessarily imply a lack of understanding, but can be attributed to a variety of reasons, such as lack of context or incomplete information. Something that happens less with humans because we live in the real world and therefore have a greater understanding of context.
Hinton also highlighted that both we and these language models suffer from confabulation, a phenomenon in which memory can betray us and produce false information. For AI, this behavior is usually called hallucination, while for us… well, it happens when we are remembering something wrong, basically.
In a nutshell, according to Hinton, the models are wrong, but there is not too much distance between us and them (a radically different opinion from other researchers). In addition, he notes that the memory capacity of such models can exceed the biological one, leading to surprising and sometimes unexpected results.
Important issues, on paper
The topics of fairness, explainability and D&I (with a focus on so-called “low resource languages”) were the golden geese of the conference. The vast majority of publications, on paper, dealt with these issues — e.g. how to leverage a certain technology in languages that are underrepresented in the original training data — but the reality of facts sings a different tune.
The issue of fairness, or equity, is of paramount importance when it comes to developing artificial intelligence models so that they do not perpetuate biases or discrimination present in the training data. Explainability is crucial to understanding how such models make decisions and ensuring their transparency. Diversity and inclusion (D&I) are key principles to be applied in both the development and use of these technologies to ensure that they are accessible and useful to a wide range of communities and cultures. Attention to languages whose open-source resources are limited is also a topic of great importance, as many languages do not enjoy the same attention and availability of resources as mainstream languages, and this can lead to disadvantages in access to language technologies.
Beyond the academic setting of the conference, one wonders whether these issues are actually addressed in reality. How much attention do companies place on these issues? And if these issues are pitted against system performance, what do they favor?
In other words,
Is it preferable for a company to have a transparent and clear system with lower performance or one that achieves maximum accuracy?
Environmental issue in only worth one workshop out of twenty-two
Large Language Models (LLMs) such as ChatGPT, while being very powerful language processing tools, present non-negligible environmental challenges. Training these models requires enormous computational resources, often supported by large clusters that consume a high amount of energy. This energy consumption, unless derived from renewable sources, can contribute significantly to global CO2 emissions. On one hand, the industry is recognizing these impacts and taking steps to mitigate this issue. Actions that can be taken to reduce impacts include adopting more efficient algorithms and reducing the size of models without compromising their effectiveness.
On the other hand, few of the accepted publications (including papers and workshops) mention this issue; even fewer consider measuring its own impact an appropriate information to put into the article, or something that is necessary to mention in order to be published. Truth be told, this is more of an engineering problem rather than a linguistic one. Nonetheless, the environmental issue is so pressing that these distinctions feel more like an excuse than a justification.
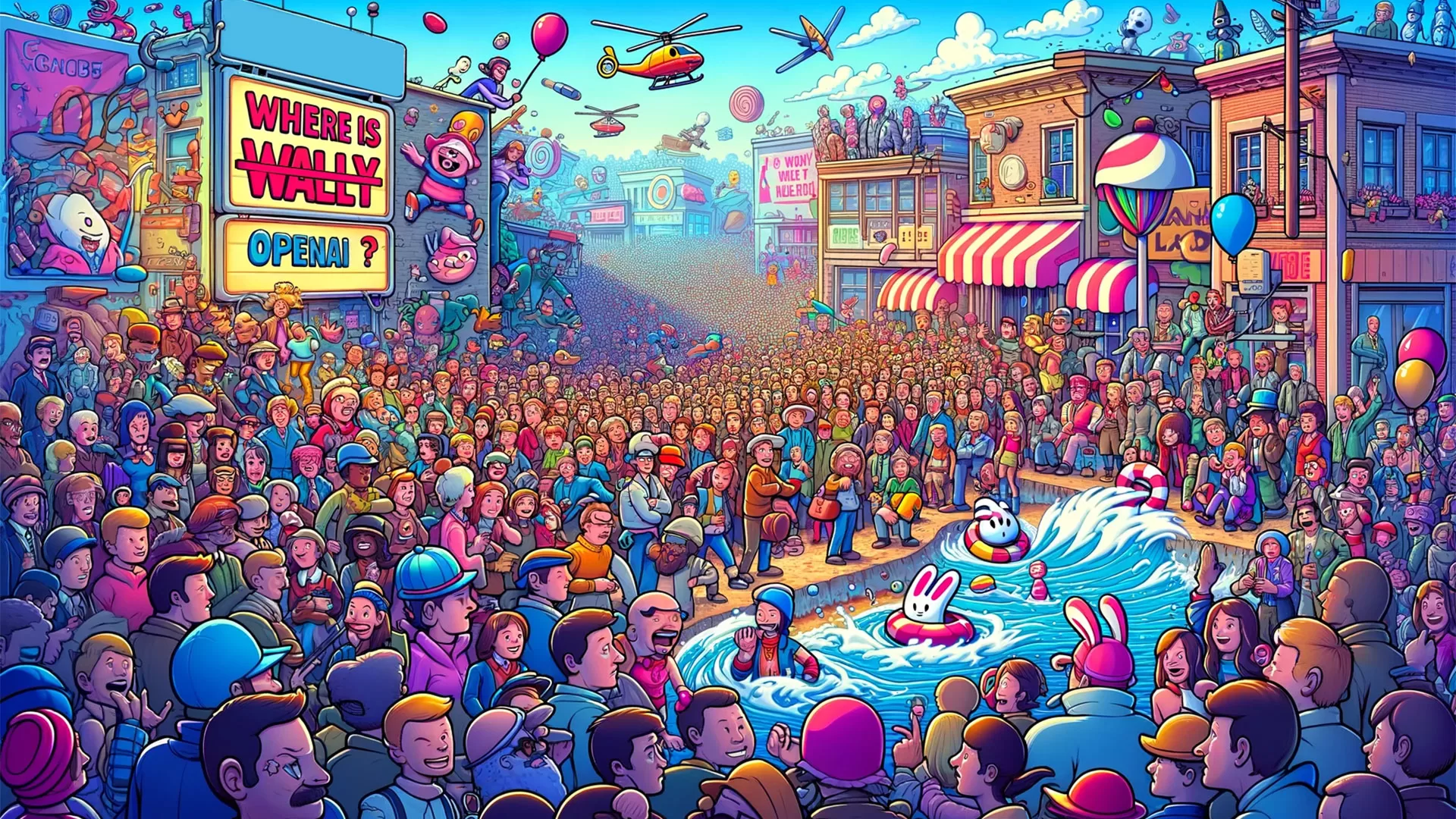
Artwork by MAIZE
Where is OpenAI?
ACL is not a strict academic conference, as companies often take the lead with excellent publications. OpenAI first started as a research organization with the goal of promoting and developing artificial intelligence for the benefit of all humankind. To date, OpenAI has left its non-profit status, becoming in effect a for-profit subsidiary company. Although its primary goal is to do pioneering research on artificial general intelligence (the hypothetical ability of an intelligent agent to understand or learn any intellectual task that a human can perform), OpenAI has not submitted any work to ACL — and mind, the conference took place before the recent turmoils that impacted the internal organization of the company.
However, many publications do deal with OpenAI’s products: ChatGPT and its “fellows” are considered competitors (comparison models to the methodology presented in the article) by several authors and they get mentioned in most of the speeches (especially those focused on the topics described above).
The conference is known for its strong opinions on reproducibility, which requires the authors, in addition to a detailed description of the proposed system, to share everything necessary for anyone to achieve the same results, i.e. sharing tools such as source code, trained models, and data. On the contrary, a great many aspects of OpenAI’s products are not known, let alone reproducible.
The open/closed source debate (i.e. the release or non-release of software components) within the community is very heated. There was even a dedicated talk during the conference with people from universities or companies that make open-source their business. The point is that GPT is the first-of-its-kind language model that from a research perspective is incredibly interesting, given the impacts it will be able to generate; an open discussion about its components and future development would have really made a difference, a singular voice that could have significantly contributed to the debate. Despite this, OpenAI was not present — nor at the debate, nor with any paper. For the first time, Wally was actually missing in the picture.
In conclusion
The ACL 2023 conference in Toronto was a major event in the field of NLP, where companies and researchers from around the world gathered to discuss the latest advances in language research. However, it seems that some crucial points of the discussion went missing, starting from the superficial approach adopted towards environmental and fairness issues, to the complete absence of one of the major players of the field of AI.
As a researcher, my instinct is to doubt, so that I can form hypotheses and then check them against reality. After the conference, some questions naturally popped into my mind:
How might the evolution of AI-based language models affect our understanding of human nature and our own intelligence?
What are the long-term implications of the widespread use of large language models on the social, cultural, and economic dynamics of society?
How can companies balance the pursuit of increasingly advanced AI models with the importance of ensuring transparency and fairness in their systems?
‘Til the next conference.