A bumpy transition into tomorrow
AI offers substantial benefits to humans, but we must exercise caution due to its potential to generate deceptive and entirely fabricated realities easily
by Vittorio Di Tomaso
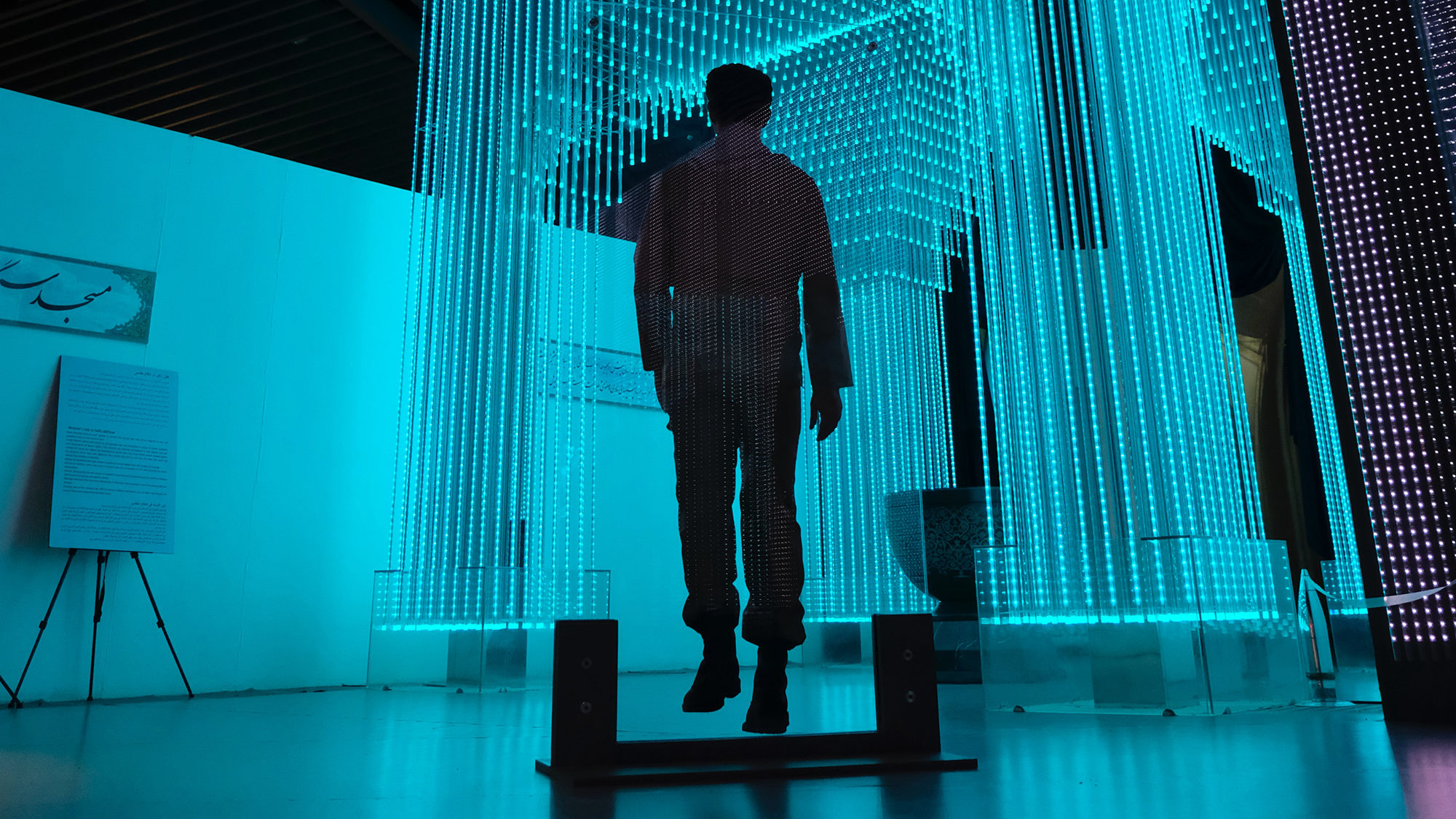
Unsplash
<span style="font-weight: 400;">A few years ago, a 30-year old researcher named Ian Goodfellow became a star in the artificial intelligence and machine learning community for inventing a novel method for training deep neural networks called Generative Adversarial Networks, or GANs. Neural networks are the backbone of machine learning because, as we discovered over the last few years, they are amazingly good learners: given sets of labeled examples, they understand the representation of the patterns underlying the data that enables them to also make sense (typically classify) of a new set of data of the same kind.
For example, if we show a network a large enough collection of pictures of animals properly labeled as cats, dogs, or okapi, after a while, the network will learn to classify an even larger set of never-seen-before pictures of animals. This ability is rapidly changing the world, making AI a transformative technology in businesses and even people’s lives. The difficulty with this approach is that networks, to learn effectively, need a lot of labeled data (this is called “supervised learning”) and that data is not always available—even when labeled data is available, there are problems relating to labeling errors and biases.
Mr. Goodfellow partially overcame the we-need-data bottleneck by inventing a method for creating new, synthetic data that is virtually indistinguishable from the original, with the idea of making two neural networks work in tandem. One network learns about a data set and generates examples, while the second tries to tell whether those data points were real or fake, allowing the first to tweak its parameters in an effort to improve.
The two networks are locked in a competition: one of them creates, for example, a picture of a cat that does not exist and tries to fool the other one into believing that the picture really represents a cat (even though we stress that the picture is not an actual photograph of a living cat, but a set of pixels randomly generated by the first network). After a while, the generator will learn how to trick the discriminator and become able to create data that is synthetic but looks real.
This dueling-neural-network approach has vastly improved learning from unlabeled data. GANs can already perform some dazzling tricks. As mentioned, we have methods that can generate images of everything, from people to cats to cars that look like pictures of real things, but that are actually fake. But AI now also allows us to realistically swap the face of someone in a video with that of someone else; generate text that no human has ever written, (even mimicking the style of a specific author); or even explore a set of possibilities and find something new, for example identifying the winning move in a game or the folding of proteins.
The metaverse is the post-pandemic version of a techno-utopia, where technology helps humans achieve their potential while digital ecosystems make a profit.
Mr. Goodfellow was heralded as the man who gave artificial intelligence a form of imagination. He went to work at Google, then Open AI (a Microsoft-backed research outfit), and then Apple, where he is director of machine learning in the aptly named “Special Projects Group”. In a 2018 interview in the Lex Fridman AI podcast, Mr. Goodfellow said that he was convinced that “20 years from now, people will mostly understand that you shouldn’t believe something is real just because you saw a video of it”. Indeed, using the generative method, we can already create new people, new stories, and even new scientific theories. We can basically create anything in the digital domain.
New technologies like GANs (and other generative methods) are quickly moving from the realm of science fiction to everyday life—just as everyday life inches closer to science fiction. And nothing is more “science fiction” than the metaverse, the next iteration of the Internet, where social networks meet virtual reality in a massive shared experience. The metaverse, as described by its proponents such as Meta (the company formerly known as Facebook), is a benign environment, where people can experience a virtual life that is even better, fuller, and happier than real life.
The metaverse is the post-pandemic version of a techno-utopia, where technology helps humans achieve their potential while digital ecosystems make a profit. But the metaverse as described by cyberpunk writers in the early ‘80s, when the Internet was an infant and a decade before the creation of the world wide web, was a much darker place. William Gibson’s cyberspace, “a mass consensual hallucination of computer networks,” was populated by marginalized, alienated losers living on the edge of a dystopian society impacted by rapid technological change.
Four decades after Neuromancer (Gibson’s most famous novel, published in 1984), we are trying (and failing) to adapt to a public discourse that is rife with cyber threats, forgeries, fake news, and all the other digital garbage that increasingly fills our cyberspace. We cannot avoid the question: What will happen when we finally realize that there can be very realistic digital artifacts (text, images, sounds) that are not real? The transition into the metaverse, thanks to inventors like Mr. Goodfellow, will be bumpier than most techno-optimists predict. And, as usual, the best—or the worst, depending on how you look at it—is yet to come.