What is the AI black box problem?
We do not fully understand why the algorithms behind AI act the way they, and this is a problem which we can't ignore.
by MAIZE
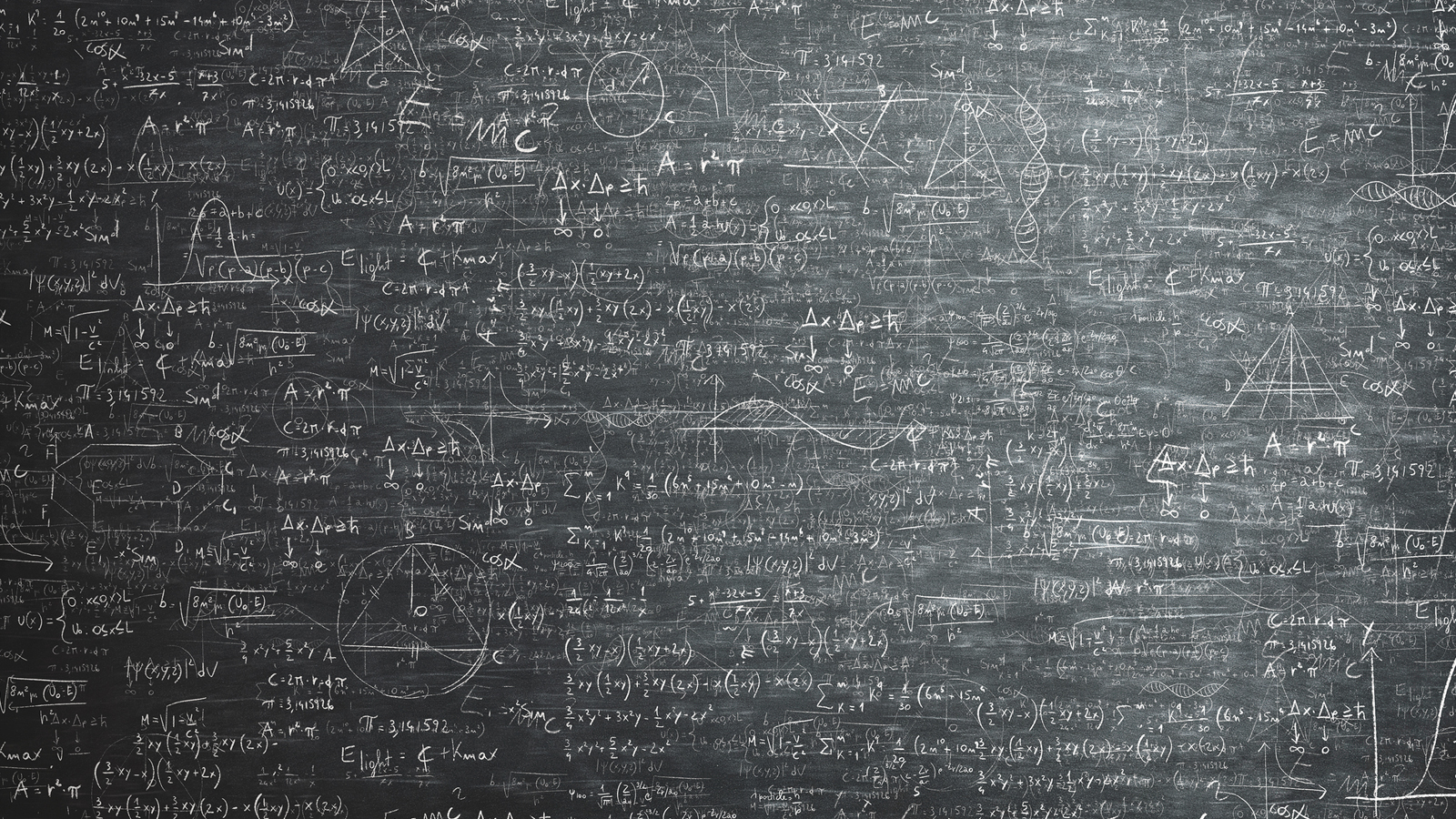
Four years ago, 18-year-old Brisha Borden and a friend spotted an unlocked bicycle in the street on their way to pick up a family relative from school. They were late and, as teenagers often do, acted by jumping on the bicycle and cycling down the street. Immediately, a woman came running down the road and informed the two girls that the bike belonged to her children, the girls returned the bicycle but it was too late – the police had been called, the girls were arrested and charged with burglary and petty theft for the items – a total of $80.
A year before, one Vernon Prater, 41 years old at the time, was picked up for shoplifting $86.35 worth of tools from a nearby Home Depot store. This was not Prater’s first rodeo: he had already been convicted of armed robbery and attempted armed robbery (for which he served five years in prison) in addition to another armed robbery charge in the past. Borden had a record, too, but it was for misdemeanours committed when she was a juvenile.
You may have heard this story before, as it was brought to light by ProPublica’s award-winning report Machine Bias back in 2016. If you haven’t, you are probably wondering why these cases are worthy of a mention. What’s so odd about being arrested for criminal activity as, unfortunate, and ambiguous as the circumstances may be?
The reason is that this story has a strange conclusion with sinister consequences. When the two were in jail, a computer programme was tasked with predicting the likelihood of each committing crimes in the future. The result? Borden, who is black, was rated as a high risk. Prater, who is white, was rated as a low risk. Two years later, Prater was serving an eight-year prison sentence for breaking and entering into a warehouse, stealing thousands of dollars worth of electronics in the process. Borden, on the other hand, committed no more crimes, the computer programme, and in particular, its algorithms, had gotten it completely wrong.
When one makes a wrong decision in life, or perhaps comes to the wrong conclusions, more often then not, through self-reflection and reevaluation, the individual concerned can reassess what went wrong, how to improve in the future and grow as a person. This is not the case with many of today’s algorithms, they act as a ‘black box’, shut away from the world wherein we can only assess the outputs of the inputs we feed them, with no explanation given as to why they act the way they do.
Today, we put a lot of faith in these faceless algorithms. We may not be able to see them, but we know they are there and, most importantly, we believe them to be forces for good. Automated algorithms show us the most relevant products to our interests, guide us through cities, power the searches that answer our queries and even determine where we deploy our police forces. However, our deal, based on good faith, is beginning to look more and more Faustian by the day as we surrender more and more of our data to entities which may be less enlightened than we once assumed.
Back in 2015, software engineer Jacky Alciné pointed out that the image recognition algorithms in Google Photos were classifying his black friends as “gorillas.” Three years later in 2018, Google ‘fixed’ its racist algorithm by removing gorillas completely from its image-labelling tech. This may, of course, be down to Google not putting any resources into fixing this, but when dealing with such a highly sensitive area such as race, and with as progressive a company as Google, it seems reasonable to believe that the reason they did not solve the problem was because they were unable to understand why the problem was occurring in the first place.
Algorithms then, run the risk of reinvigorating historical discrimination, encoding and reinforcing it once more into our societies. The fact that Google, seen as a forerunner in the AI-sphere, cannot overcome such a problem illustrates the deep complexities of machine learning and algorithms and how little we understand them. There is a saying in the coding world: if you input garbage you will get garbage. If you input bias, even if unconsciously, you will output bias at the other end.
Frank Pasquale, author of The Black Box Society: The Secret Algorithms That Control Money and Information, and quoted in Ed Finn’s What Algorithms Want, states that: “The automated systems claim to evaluate all the individuals in the same way, avoiding discrimination” however “prejudices and human values are incorporated into every comma of the development phase. Computerization can simply transfer discrimination further upstream”. So what can we do to mitigate these malgorithms? How can we shine a light into these black boxes and reveal their secrets?
Currently, it is almost impossible to determine whether or not an algorithm is fair, as in many cases, they are simply too complex to fathom. Furthermore, they are often considered as proprietary information with laws in place that protect their owners from sharing the intricacies of the programmes they use.
In 2016, Wisconsin’s highest court denied a man’s request to review the inner working of COMPAS, a law enforcement algorithm. The man in question, Eric. L. Loomis was sentenced to 6 years in prison after being deemed high-risk by the algorithm. Loomis contests that his right to due process was violated by the judge’s reliance on an opaque algorithm. In an attempt to understand how states use scoring in their criminal justice systems, two law professors probed the algorithms for a year, with the only discovery being that this information is well hidden behind staunch nondisclosure agreements.
But there is hope: a team of international researchers recently taught AI to justify its reasoning and point to evidence when it makes a decision. This form of AI is able to describe, through text, it is reasoning behind its conclusions and is one of few developments in the progress of ‘Explainable AI’. According to the teams recently published white paper, this is the first time an AI system has been created that can explain itself in two different ways. The model is the first “to be capable of providing natural language justifications of decisions as well as pointing to the evidence in an image.”
The researchers developed the AI to answer plain language queries about images. It can answer questions about objects and actions in a given scene and provide answers which require the intelligence of a nine-year-old child. It doesn’t always get the answers right (it mistook someone vacuum cleaning a room for painting one for example) but that is precisely why this development is important. It gives us a glimpse and understanding of why it got the question wrong.
It is not only in the lab where there is growing concern about the unintended consequences of AI. Last April, Harvard Kennedy School’s Belfer Center for Science and International Affairs and Bank of America announced the formation of The Council on the Responsible Use of Artificial Intelligence (AI). A new effort to address critical questions surrounding this far-reaching and rapidly evolving application for data and technology, the Council is focused on issues ranging from privacy, the workforce, rights, justice and equality as well as transparency.
“It is difficult to overstate AI’s potential impact on society,” said Ash Carter, Director of the Belfer Center and former Secretary of Defense (2015-2017). “The Council will leverage Harvard’s unmatched convening power to help ensure that this impact is overwhelmingly on the side of public good.” As more and more money pours into AI, it is paramount that we understand the processes behind the processes, and build awareness of the risks misunderstood algorithms bring.
Even so, until this technology is finessed, many will continue to find their lives determined by the damning sentences and evaluations these black box algorithms inflict upon them. As we have seen, it is almost impossible for citizens to bring a case against the algorithms’ creators, and these unseen forces continue to remain unaccountable for the consequences of their actions. Algorithms are certainly one of the keys to the world of tomorrow, but what that world looks like, and what values it is built upon, remains to be seen.