AI Integrations are strategic design challenges
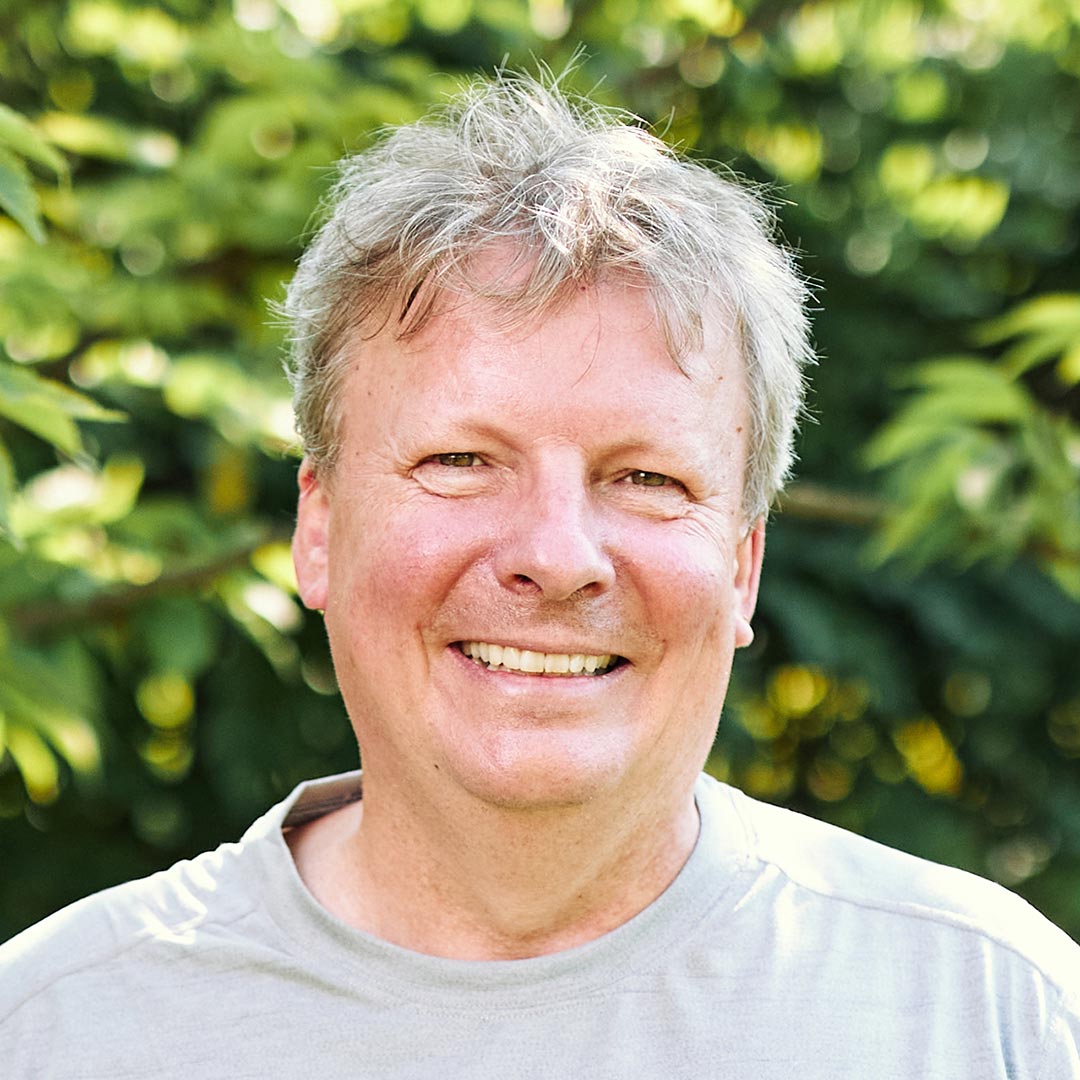
Christian Schneider
Partner, MAIZE
Ventures, products, and services
2024
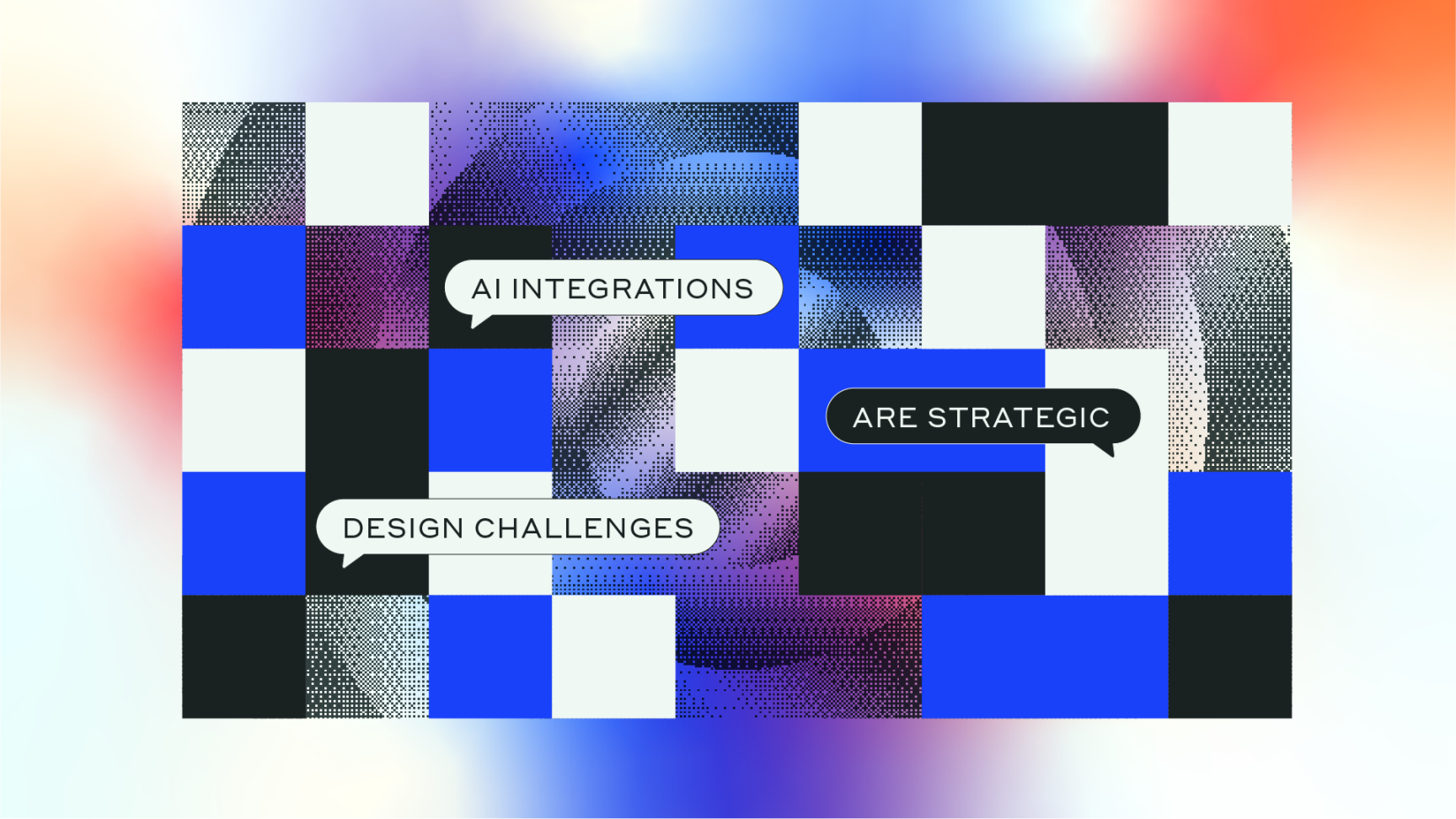
We have seen AI stocks soaring high but encounter hesitations and lower expectations on the ROI when proposing to integrate AI in organizational settings that frequently need more infrastructure, data availability or quality, and expertise. Even the dot.com hype created high expectations, a bursting bubble, followed by years of designing and integrating web-based applications into the way we work and live, finally changing companies and economies in almost every regard. Approaches and processes for design-driven innovation have evolved with countless precedents of previous tech integrations.
This essay outlines how the ongoing AI spring:
- Impacts the way we design and extends the reach to whom we design with.
- Requires designing AI integrations in services, processes, and systems.
- Enriches the design process (?).
1. AI IMPACTS ON DESIGN
“Machine intelligence is the last invention that humanity will ever need to make,” or “Success in creating AI would be the biggest event in human history. Unfortunately, it might also be the last”. There are numerous predictions of this kind, and some of the voices are amongst the most influential in this world.
Is it that big, or just a superhuman marketing campaign? Asking ChatGPT on catastrophic visions of AI impacts, it says that “it can become super-intelligent, surpassing human intelligence and capabilities, which raises concerns (of the humans) about losing control.”
AI is often seen as the most significant revolution after industrialization. In industrial production, the most important innovation for the workforce was the red button, which allowed workers to stop the machines they were operating. This button decreased health issues and increased the well-being of workers who learned to use even more complex machines.
So, if we don’t want to lose control, which seems to be the basis of all fears, we can learn from history that we should make sure that we can always turn that thing off. Otherwise, there is no plausible reason not to tinker around and make use of such promising tech developments.
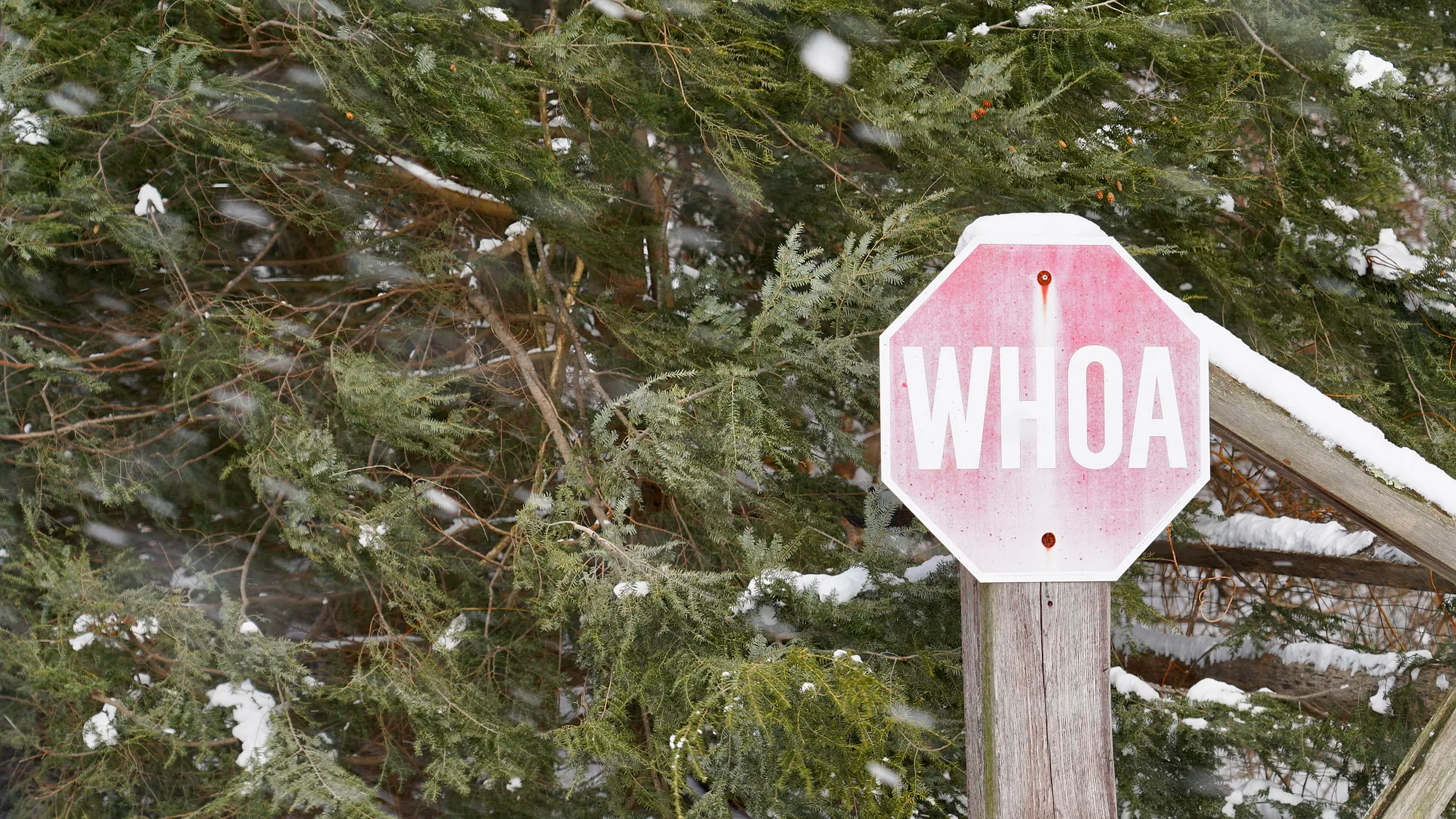
A total democratization of design
With ChatGPT, a successful attempt was made to reach the masses. Further tools followed, and they allowed to create without having a specific skill, such as visualization, or in-depth software knowledge.
With access to those tools, we can overcome the privilege of trained skills. Creating with words has few limits; maybe, one day, we will be able to create just with our thoughts. Imagination is of everyone.
While hardly anyone asked how inclusive the metaverse would be — or who exactly we can categorize with our data analysis — free access to AI tools can be for many, everyone owning a computer with an internet connection.
By increasing the number of creators, the world could see new perspectives, depending on what will be recognized and integrated if we design spaces to co-design, keeping an eye on how manipulative and exclusive our reach will be and with which criteria we will codify and filter the inputs we receive.
A crucial design challenge remains our attention to diversity and inclusion and the ongoing effort to create spaces that see diversity as a potential for innovation. The risk would be that with the sheer quantity that theoretically is possible to reach, it all flattens to one homogenous grey.
What are our expectations here? Maybe not necessarily creating uniqueness, but examining common perception, collective consciousness, and patterns of the status quo of needed and desired innovations. In theory, we can bring together and orchestrate endless inputs.
A new need for the new
Increasing access to creator tools has precedents. Music had this, but if I play current house music on Spotify, most of it sounds pretty similar to me. Which makes it even more evident how difficult it is to create new, good music, to produce and distribute it, and for listeners to find it.
It is also true that after Nokia became the biggest producer of cameras a couple of decades back and photography was made accessible to so many through the mobile phone, allowing people to take pictures of high technical qualities, we still admire a low-resolution black and white photograph from Henri Cartier-Bresson; or projects like “shooting back” from the Israeli Human Rights Group B’Tselem (that gave video cameras to Palestinians to record their everyday lives).
Alessandro Mendini once said: “Everything had been designed — no new form is possible.” (Alchimia, 1988) The Alchimia movement created contrasting arrangements of different textures, styles, materials, and forms, bringing all imaginable design styles together and re-arranging what had been designed already.
I was visiting Alessandro Mendini’s Studio in 1989. In a conversation, Mendini said that at his newly formed Domus Academy, education would not focus on the skill set needed to design but on elaborating on why we design something. That was a few years back, but frontal teaching expecting students to absorb their areas of interest like machines can still be found in so many Universities.
With advancing AI, we don’t need to train our brains to store and combine knowledge, but to create context to apply knowledge. Universities will have to rethink how they teach what. Beyond that, they can keep their traditions as a backup in case of a system failure — just as I kept my vinyl.
The victory of the unpublished
In Wim Wenders’ movie “Lisbon Story”, the fictive filmmaker Friedrich is convinced that anything captured in pictures or films vanishes with the viewing. In an abandoned cinema, he stores recorded but unseen movies of a Lisbon that no longer exists.
It’s a concept that made it through the centuries, “If only you would keep silent and let that be your wisdom” (Job, Chapter 13, Verse 5) and “If you can bear to hear the truth you’ve spoken, twisted by knaves to make a trap for fools” (“If” by Rudyard Kipling). Today, there is a paradox: If we create something new, we publish it to claim ownership.
It is still the most efficient protection, as IP challenges evolve much faster than legal regulations. However, if we publish something, it can get caught by AI, integrated, flattened, or misinterpreted. The unpublished new is instead pure, unique. Should we keep it in an abandoned cinema and refrain from implementing and sharing it? Who owns the design created by the AI? Is it the AI? the developer? the artists whose data the AI is trained from? the user?
Balancing a desired broad web reach with a new, limited, company-specific area will be a strategic design task for corporations. Corporations and groups will cultivate their restricted platforms, safe spaces to share their content. Places where some are part of a group — like villages or nations — just not one geographically defined. The scope is not to reach as many people as possible, but to distinguish and keep an identity unique.
AI can help us see ourselves in one world, our individuality, and our common problems. By bringing together information, insights, and different approaches, we can find better, more complete solutions for global issues. New creators, uniqueness, attribution, manipulations, and ethical concerns are construction sites that a wider use of AI brings with it.
Besides those evolutionary challenges, strategic design should be a driver for understanding, evaluating, and supporting reasons for AI integrations, followed by strategies for appropriate implementation processes.
2. DESIGN FOR AI INTEGRATIONS
A humble consideration of so much in the years to come
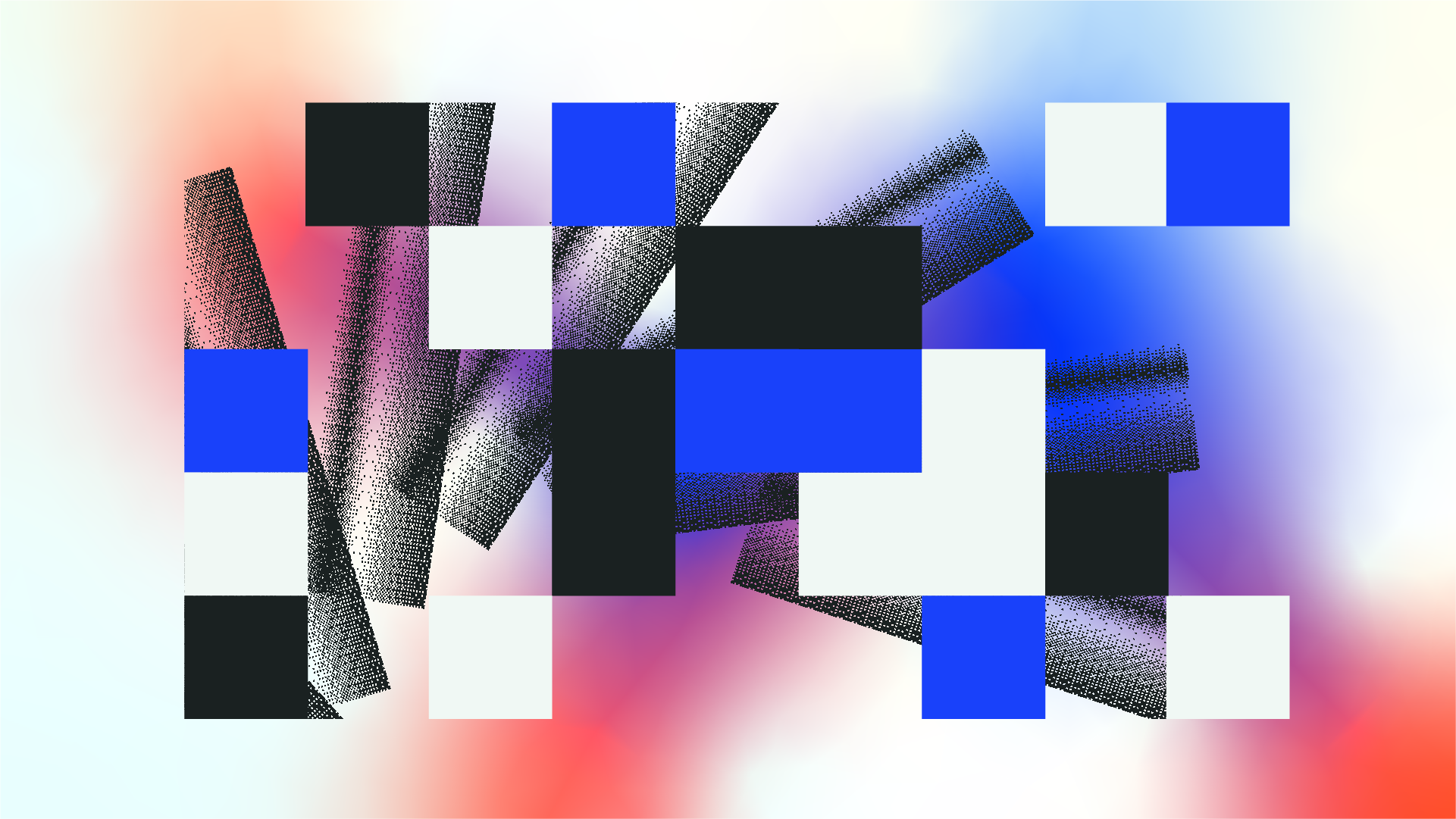
Good design
The most obvious design challenge would be to help many of those 150,000 GPTs that made it into the GPT Store. They clearly need support from traditional design disciplines, to overcome the neon obsession, the sci-fi identity, and the gimmicky, cartoonish, fun appearance.
We can start all over and suggest investing in good design to the benefit of the business. Then, we can continue improving the interaction design, etc.
But timelines are short here, as the changing platforms and the kinds of interaction will change the entire appearance of a given model before work on it can reasonably begin.
System design
As initially stated, a broad design engagement and analysis can help to see a complete picture of currently imaginable design ideas and languages, delineate design perceptions in their totality, and foresee underlying and unmatched trends.
Inside corporations, AI can recognize and enable a corporate design DNA based on the entire design output of all entities: it can capture an organization in its complexity with all its visualizations and contents that reflect design expressions to see common denominators, patterns of individual interpretations, or inconsistencies.
In particular, corporations composed of various entities in different corporate areas and locations can benefit from recognizing new design practices and contents to align those entities better and evolve a common design language.
Organizational design
There are numerous potentials, such as optimizing workflows and processes, automating repetitive tasks, analyzing employee sentiment, and identifying skill gaps and resistance to change processes.
AI can support decision-making processes, making them transparent and fact-driven. Much of all this is theory, though, as it largely depends on the data and how to gather it. Future models may be able to do extrapolations requiring far less data — and indeed far less structured data — than we may need or expect.
There may be free (data-) food for everyone, with new AI-driven models that gather and make data available. For now, you need to do your digitalization part first to consider AI integrations, just like you need genuine ingredients to cook good food.
A practical example would be to throw together all OKRs employees are creating to see patterns, common denominators, areas which are not covered or too much aligned, where there is double the effort and where there is none — how the greater group in various locations (especially in this current period of mergers and acquisitions) functions together.
Playing with the support of AI tools might also help to understand the limitations of OKRs, show potential for improvements, and work on the matchmaking with KPIs.
Social design
AI technologies such as natural language processing, speech recognition, and computer vision can improve accessibility. We can design systems that provide personalized support and assistance to individuals facing social or economic challenges.
Chatbots and virtual assistants offer information, guidance, and resources related to healthcare, employment, education, and social services. AI can improve these interactions by helping people navigate complex systems and access the support they need.
Also, AI algorithms can analyze large volumes of data from various sources to identify patterns, trends, and insights relevant to social issues such as poverty, healthcare, and education. When gathered, this information can finally provide information for appropriate policymaking.
As there is potential to access information for many, an unclear origin of information will impact societies when everyone becomes skeptical about everything, knowing that anything we see might not be accurate and authentic or exist without ownership.
Evolving AI might have a side effect; maybe it will turn upside down the current power of false information in social media — as we realize that everything might be manipulated, we might get back to accredited and professional sources.
Future scope scenarios
Future scope scenarios are clearly a design challenge. AI fuels our fantasy with all imaginable applications, like Danno, the disaster assistant, an autonomous search and rescue member.
Someone on the related LinkedIn post further asked about the rights of our new non-biological colleagues, which adds a perspective on ethical concerns related to AI.
As for the new virtual world, at the end of February 2024, the value of Nvidia rose in only one day by $277 billion, more than any corporation ever before, and at the time of publication, the firm is worth over $3 trillion, up there with the two most valuable companies (Apple and Microsoft) and overtaking the entire German stock market in value.
The relation between NVIDIA Omniverse and AI lies in the use of artificial intelligence techniques to enhance and optimize 3D content creation, simulation, and rendering. Particular attention in the gaming industry lies on non-playing characters (NPC), which apparently can self-train and self-evolve to some extent.
With the potential earnings in this industry, expensive robots will also decrease costs and grow in learning capacities. Technology might eventually solve a problem it created: solitude.
3. AI FOR THE DESIGN PROCESS
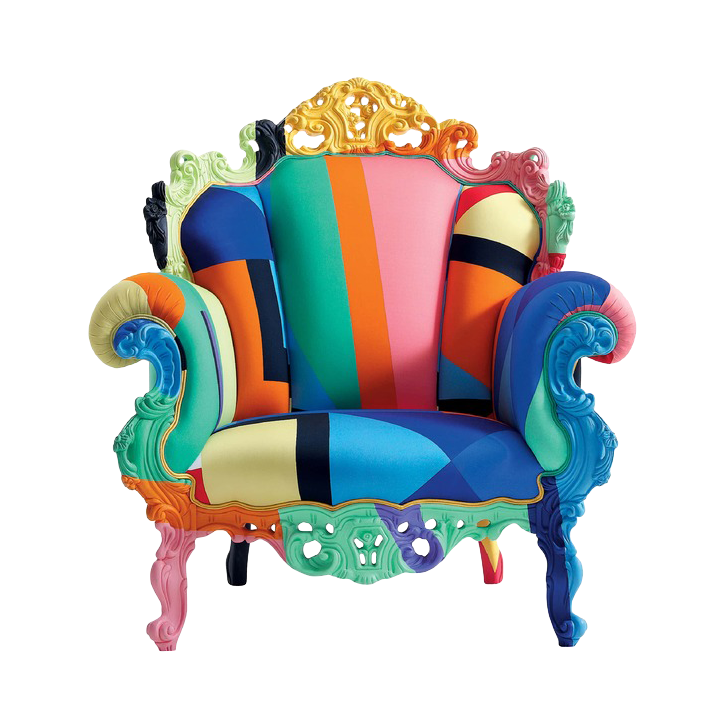
That much for design research
For now, it seems evident that we cannot “hand over” design research to AI and a set of GPTs. In our process, we constantly open up and do not work toward a defined goal as they do in scientific research. We aim to extend the spectrum of possibilities and do not narrow it down to one solution. We need this open approach to leave space for innovation “to happen”.
We define the area that requires new solutions, but we don’t know what the end result of the design process will be; at times, we don’t even know all that we need to solve. The nature of design research is that, this sort of continuous vagueness is hard for us humans, and it is logically impossible for machines.
It remains to be seen if self-learning systems can develop and apply open, organic processes, evolve into some kind of “creative artificial intelligence,” and unveil opportunities following design research processes.
Already today AI can be of great assistance, though. With our quantitative explorations, we investigate a research direction — i.e. an area in need of innovation — with data, features, and texts of all kinds and from various sources; information that either proves or questions the validity of the research questions being asked.
AI can help us process vast sources of text and data, and it can help us to structure this information, map it all out to get a clear picture of what we are looking at, and reveal blank spots.
The rule of thumb in design research is to challenge our assumptions and any information we encounter. We aim to find and integrate non-obvious and contrasting information, cross-fertilize with information relating to different or analogous areas, and change perspective to see things differently.
We play with data to get to see above and beyond the status quo. We don’t want to prove anything in this process; we want to create space for diverse input and seek the unexpected. We need two true anarchists: chance and coincidence. We must be there and catch them when they appear, which is always when we don’t expect them to.
As far as the more structured surveys are concerned, it is still a design challenge to create survey platforms that build on AI tools. It can play a pivotal role in streamlining data collection processes through automation, diminishing the reliance on manual input, and utilizing various channels such as online forms and voice interactions.
Theoretically, AI can enable personalized survey experiences tailored to individual characteristics and preferences. Such wealth of information could provide deeper insight into customer satisfaction, employee engagement, or public opinion.
We co-create the content with the AI, then leave the operational part to the AI, reducing time and resource requirements and resulting in cost savings and quicker turnaround times for obtaining insights. It’s a lot of work to get all that started, though.
Surveys are somewhat in between quantitative and qualitative research. They are not enough to make a point with additional data and are not helpful in getting under the skin of people, the most precious part of design research.
Besides our decennial experience using AI and NLP to extract knowledge and support innovation projects with language-related data, our explorative Crew Projects at MAIZE created an AI chatbot to conduct interviews and generate follow-up questions and reactions based on user responses.
While lacking empathy in crucial emotional moments, the privacy afforded by the interviewee’s environment did impact their willingness to share. Prompt design, alongside human supervision, remained crucial, particularly when the AI randomly filled blank spots. Further improvements like voice recognition, tone detection, and image interaction await follow-up explorations. (Am(AI)zing Brain Crew)
In the design research process, there is a distinction between Interviews and open forms of conversations, which traditionally derive from observations. We interview service providers but observe service receivers, producers but observe users, an employer but observe employees, etc.
With observations and open conversations, we want to challenge (and not complement) what we have learned from quantitative information with qualitative insight. Even if numbers define an area we are working on, the underlying sentiment can still be very different.
For instance, data can tell us that a company is on track with expectations in all areas. This could still be perceived as widespread fear amongst employees about their future, and vice versa — wrong numbers and positive, engaged employees.
The power of human
We observe people in their environments and make them feel safe so they say what they think and do , not what they think they should say. Instead of a set of questions, we formulate “teasers” that push people to comment freely in those directions. The art is to make people feel comfortable to share a piece of themselves.
Getting to that point requires skills in the domains of psychologists and anthropologists. It can take time and various attempts until people open up, but the insights are priceless once they do.
If we capture the heartfelt, real frustrations of eight people using a certain service or function, for instance, we don’t need to ask a ninth person. We learned and can refine accordingly. We might not get these real insights when surveying a thousand people.
We do need evolution in design research to overcome the dominance of established design and business cultures when driving these processes, which can be manipulative, starting by choosing people for these open conversations, locations, and our presence during these observations.
Instead, we should repeat design processes by local designers in various places. We need widespread knowledge of the design process and access to current and not second-hand technology to capture this potential.
Testing otherness
We can use AI tools to overcome even subconscious bias and increase the spectrum of personas with the ambition to avoid categorizations — making them so vast that they vanish. With the help of AI, we can become someone else (online), which is another human desire beyond our control.
If you are a male permanent resident workforce, become a female immigrant and apply for a job, for instance. Build your virtual AI presence based on your skills and experiences, not outer appearance and bureaucratic categorization, and become active. You desire, the AI suggests, you choose and execute.
Give yourself in other hands, like a trade-in for no cost. We can create and test almost anything — what remains is the content, who we are beyond appearance and categorizations.
More for strategy design
With AlphaGo, AI played itself millions and millions of times until it got so intelligent that it did not only know what to do in any given situation, it also came up with strategies that no one would have ever thought of.
That worked for a game; but in real life there are more moving parts, and the rules change constantly. Still, strategy design in development processes is not about “being strategic,” but designing a plan to get a concept to implementation.
AI can be used in assessment tools that capture all available data, anticipate roadblocks, analyze risks, and evidence needed refinements.
Also, the potential of AI developments seems to be open here. The principles of gradient descent (implemented as optimization algorithms) can be compared to “changing perspectives” to “find smallest common denominators” in strategic design, that give orientation to our iterative, non-linear design process.
It is fascinating to study these functions as a design expert, as there is potential to convey principles of design driving innovation to AI tech experts. Below is a short overview of how current AI technology can assist in the design of a strategic plan.
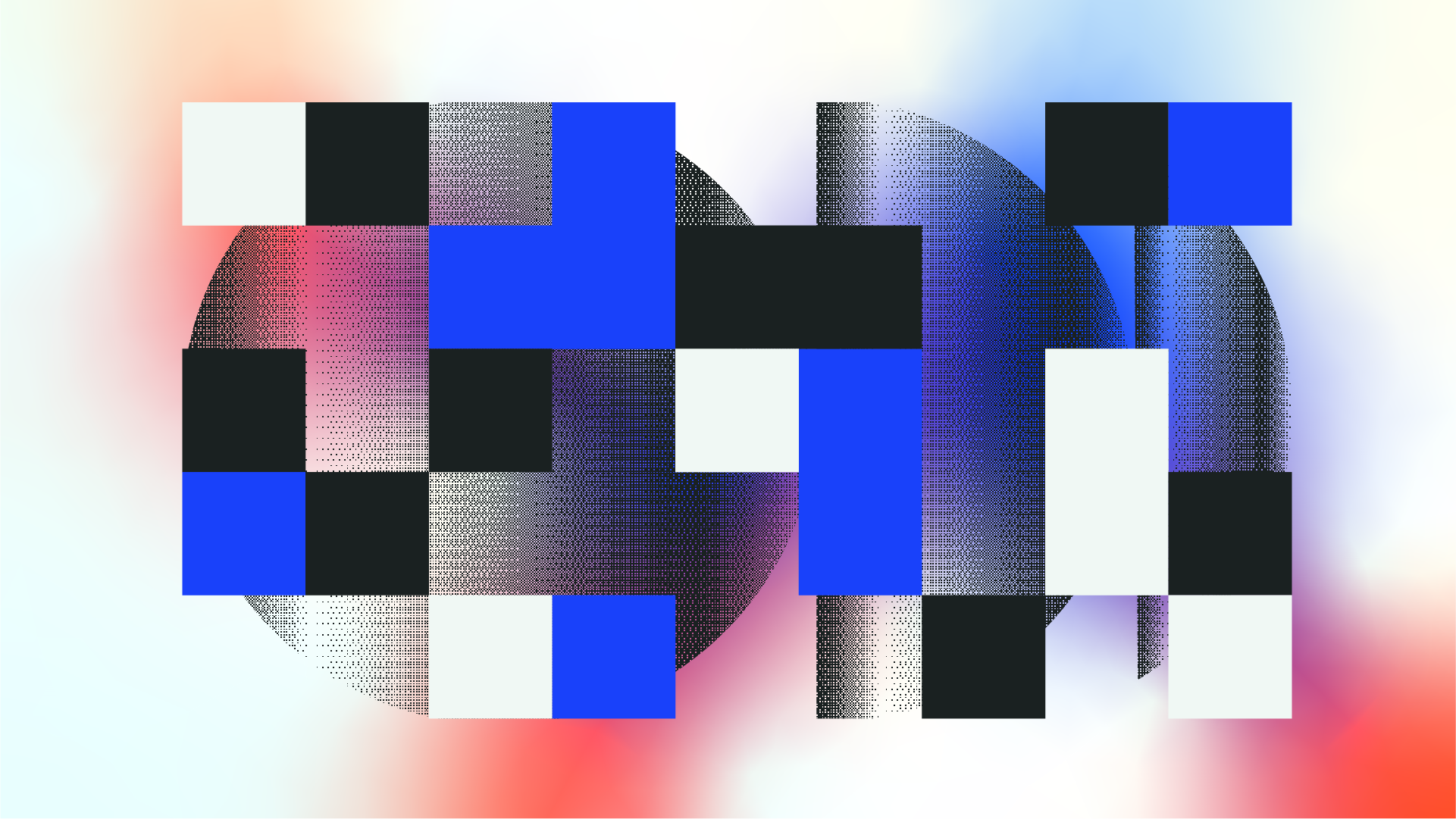
Function trees
When individuating core functions of a product, service, or process that respond to our design research findings, we opt to see a complete picture even if we cannot lay out entire function trees.
Here, machine intelligence can help to scan and fit together a variety of smaller functions to correspond to the desired core functions, values, and requirements, the tedious job of elaborating in detail what the big picture shows.
Any strategy development, therefore, becomes more efficient, complete, and safe in the way that obstacles on lower levels can be foreseen and addressed, the impact on core functions calculated and eventually solved.
Roadmaps
AI algorithms can help optimize the allocation of resources, such as budget, people power, and time, and help in finding the most efficient pathways to achieving strategic objectives. They can also help identify unforeseen needs and therefore avoid unnecessary delays or bottlenecks.
The art of the design process is to allow for iterative improvements. A goal of AI integration would be to enable continuous learning by analyzing feedback and performance metrics.
If we can oversee progress against strategic goals and adapt the roadmap accordingly, we can iterate and refine strategies to stay responsive in changing situations — and communicate that to stakeholders with some more credibility — that it’s the AI speaking.
Those with experience in strategy design and the guidance of all stakeholders understand the enormous value.
Development matrix
Qualitative perception is not just black or white, good or bad — recognizing and being able to determine and describe the shades of grey in between allow for progress in our development processes, which would be a significant distinction from traditional strategy developments and implementations and key to the success of design-driven innovation.
In strategy design, the development matrix is a structured framework that helps organize and understand whether the overall development is on track and aligned with values and objectives defined in the design research phase.
We design assessment tools that capture the sentiment of the perception of prototypes and development stages. Also here, AI can help automate assessments continuously over time and communicate qualitative perceptions to align all involved stakeholders, enabling evidence-based decision making with less tangible qualitative factors.
To describe various levels of qualitative perceptions and ambitions is a quite sophisticated task, if those factors aim to be new and unique — which would be another crucial requirement for success in design-driven innovation, and it may not be a task for AI to create, but to bring together all related qualitative perceptions and to structure those.
Pilots
Maybe the most imminent use of AI is for pilots to test market perceptions. You can type in a related bot anything, and it will generate an appropriate picture; and, soon videos.
The quality leaves to desire, but as we can shape our imagination with little effort, as initially described, we can also test product and service perceptions with little time and money.
You might realize that you have already seen a scenery or action, just with other faces, related products, or environments. Much has already been designed, and the AI takes what it gets — greetings from the Alchimia design movement.
AI is predicted to generate most online content, distorting the perception of human beauty. Faster and nicer. Learning from pilots, we’ll eventually have to unwind back to the real.
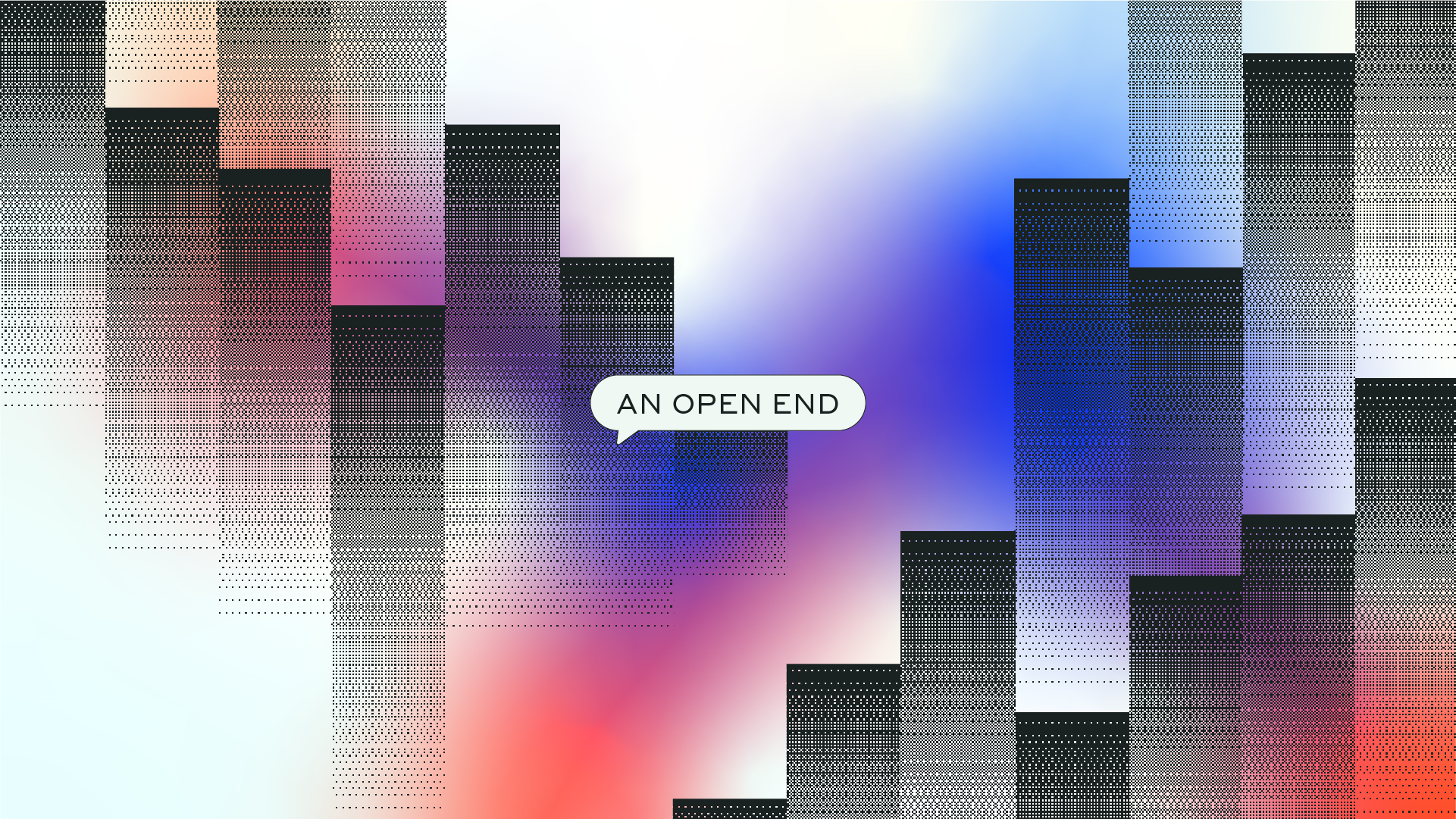
4. AN OPEN END
AI integrations in existing processes and systems are design challenges. Beyond optimizations and advanced analysis, there is open-ended potential for using AI for innovation without going as far as addressing AI incidents and hallucinations, which would be another feature.
The preceding overview merely scratches the surface of rapid developments and ongoing learning — a perpetual journey that would be better suited for a diary than a feature.
After a year of learning and codifying AI developments from a strategic design perspective, it is obvious that this is just the beginning of a long and meaningful journey.
A journey for which we should set our ambitions but travel without pre-defining opportunities, restrictions, and goals, just as we do in the design process.
Come on board if you enjoy traveling without a destination, but with a clear understanding of what you want to achieve and what you need to avoid.